14:30 - 16:00 Wake modelling and forecasting
Resource assessment


Room: Hall E
In the session there will be five presentations on wake modelling and forecasting. They will focus on the limitations in wake modelling including comparison with field data, and will move into short-term power production forecasting as well as studies of uncertainty in wind power forecast methods.
You attended this session?
Learning objectives
- Understand the latest news regarding limitations and possibilities within wake modelling approaches;
- Exemplify forecast methods and their ability to accurately predict short time ahead production output;
- Address the importance of atmospheric stability in wind power forecasting and wake modelling;
- Determine whether simple linear wake combination methods as presently used in commercial analytical software can correctly represent turbine interactions.
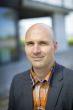
Presenter
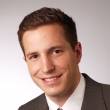
Co-authors:
Tilman Koblitz (1) F
(1) Vattenfall Energy Trading, Amsterdam, The Netherlands
Presenter's biography
Biographies are supplied directly by presenters at WindEurope Summit 2016 and are published here uneditedDr. Tilman Koblitz has a Ph.D. in CFD modelling from the Technical University of Denmark, and holds a master degree in Aerospace Engineering from the University of Stuttgart, Germany. The last 6 years he has been active in the development of forecasting and resource assessment models. His current work focusses on increasing the quality and efficiency of the forecasting system in order to optimize the marketing and sales of renewables and better handle the inherent weather risk.
Abstract
Uncertainty of wind power forecasts and optimising the use of weather intelligence
Introduction
For forecasting of renewable energy generation, the wind industry is relying on weather data that focus primarily on wind speed predictions at hub height. Additional information like the variation of meteorological variables with height or probabilistic properties of these variables cannot be provided by single point forecasts at one height. Therefore, physical processes that are crucial to the atmospheric boundary layer (ABL) and the performance of wind turbines, like for example atmospheric stability, and statistical properties like uncertainty distributions, are often ignored in operational forecast and wind resource assessment models. In order to predict the wind energy production more appropriately, especially in complex terrain, these effects should be included in such models.
Approach
The starting point of the present study is operational data from onshore & offshore wind farms in the UK, the Netherlands and Germany, with a total installed capacity of around 2GW over a period of over 2 years. Together with site-specific point & probabilistic weather data from global and mesoscale weather models (ECMWF, UKMO, HIRAM), wind speed and wind power forecast errors can be analyzed.
The aim of this work is to better understand the physical and statistical processes within the ABL relevant for short-term wind power forecasting, and ultimately to reduce forecast uncertainty. The idea is that additional weather intelligence can help to predict the varying conditions in the ABL more appropriately
Main body of abstract
The present study analyzes the error distributions of wind speed and wind power forecasts for a range of operational wind farms. SCADA data from over 600 turbines is used together with site-specific wind speed and direction forecasts at different heights and ECMWF ensemble wind speed forecasts at hub height.
As a first step, forecast error distributions from wind speed and wind power forecasts are quantified. It is shown how they vary in space and time: distributions are site-specific and depend on wind speed as well as season and weather situation. During high and low wind speeds, wind speed and wind power forecasts show asymmetric error distributions and it is shown what implications this has on forecasts models.
Secondly, it is analyzed how additional weather data can be used to characterize and reduce uncertainties. Asymmetries in probabilistic wind forecasts can be used to better assess the risk during adverse weather conditions like overwind situations. From wind speed forecasts at different heights a wind shear exponent can be derived as a proxy for atmospheric stability. The effects of different stabilities on turbine wakes and the performance of wind farms are shown. Additionally, it is shown how atmospheric stability can be linked to daily patterns in forecast error for a whole onshore portfolio in Continental Europe, induced by the diurnal cycle in the ABL.
Conclusion
The error distributions of wind speed and wind power forecasts are analyzed based on operational data from onshore & offshore wind farms in the UK, the Netherlands and Germany together with probabilistic weather forecasts. It is shown how certain forecast errors can be explained by physical processes like atmospheric stability or by statistical properties from probabilistic weather forecasts. These effects are quantified and it is shown how they can be minimized using additional weather data.
In summary the results show that the use of additional weather data helps to understand and minimize the forecast error for onshore & onshore wind farms. Suggestions are given on how to implement the additional weather data into operational forecast models.
Learning objectives
- Address the importance of atmospheric stability in wind power forecasting
- Assess the potential value of ensemble forecasts
- Analyze the error distributions of weather and power forecasts
- Suggest how additional weather data can be included in numerical models
Follow EWEA on: