14:30 - 16:00 Advanced control strategies for wind plants
Turbine technology


Room: Hall G2
Modern control design methods like non-linear model predictive control can take account of complex dynamics and actuator constraints, while making use of richer information input from advanced sensors such as LIDARs. They contribute to the coordinated control of entire wind farms while meeting new operational demands from the electricity system. The session addresses advanced state estimation methods, an important component of such controllers. You will hear about field test results from a controller using wind preview from a LIDAR sensor as well as changes in design and certification guidelines needed to account for the use of LIDARs.
You attended this session?
Learning objectives
- Advanced non-linear state estimation techniques for wind turbines that run in real time;
- Non-linear model predictive control that rung in real time and improve performances;
- A LIDAR-assisted flatness-based controller validated in field tests;
- Requirements for design and certification of wind turbines with LIDAR assisted control;
- A wind farm control framework that can mitigate wake effects and provide grid balancing services.
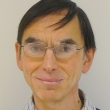
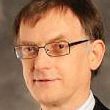
Presenter
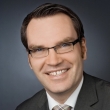
Co-authors:
Axel Schild (1) F Sebastien Gros (2) Bastian Ritter (3)
(1) IAV GmbH, Gifhorn, Germany (2) Chalmers University of Technology, Gothenburg, Sweden (3) Industrial Science GmbH, Darmstadt, Germany
Presenter's biography
Biographies are supplied directly by presenters at WindEurope Summit 2016 and are published here uneditedAxel Schild is currently working at IAV GmbH in the field of advanced automation solutions for the energy sector. In 2011, he received his PhD degree in electrical engineering from the Ruhr-University Bochum, Germany. From 2010 to 2013 he held a position as an expert for advanced process control in the German process industry. His scientific expertise covers control theory, process automation and mechatronic systems. His R&D activities concentrate on nonlinear model predictive control for renewable energy plants and energy networks.
Abstract
High-performance non-linear model predictive control for wind turbines
Introduction
With steadily increasing wind turbine sizes, there is a growing interest in alleviating structural fatigue through advanced control concepts. While nonlinear model predictive control (NMPC) is considered a strong candidate to meet this objective, it is typically rated as difficult to tune and computationally intractable on industrial embedded control hardware. Recent publications therefore promote economic NMPC (ENMPC) formulations, which, compared to classical tracking NMPC, not only improve the control-loop performance, but most importantly ease controller tuning. On the downside, such formulations seem to be computationally even more challenging, because ENMPC employs a direct measure of the plant performance in the cost function, instead of the tracking deviation of control and manipulated variables from their given references.
Approach
The presented work aims at leveraging the true potential of NMPC for improving a turbine’s operation by making use of most recent advances in optimization theory and tailored algorithms. It impressively demonstrates that NMPC is getting ready to challenge traditional PID as an industrial standard for wind turbine control in the years to come. First, a new relationship between an ENMPC formulation and its classical tracking counterpart established, which provides novel and valuable insight for the tuning of predictive turbine controllers. The paper continues by reporting so far unmatched performance and computation times of tracking and economic NMPC at representative simulation studies with the realistic 5MW FAST reference turbine simulator. Finally, the effects of model mismatches in the tower fore-aft frequency and of a limited wind speed preview horizon via LIDAR are investigated.
Main body of abstract
Against common beliefs, it is proven in simulations that predictive collective pitch control can be readily deployed on state-of-the-art embedded control hardware and is able to achieve outstanding performance with respect to balancing power production against structural tower fatigue caused by fore-aft oscillations.
Only a fairly straightforward, reasonably parametrized nonlinear prediction model is required in conjunction with a sufficiently high sampling rate. The key for the impressive control performance lies primarily in replacing the classical surrogate objective of tracking a steady-state-optimal generator speed by the true economic objective, which is maximum energy harvesting. This yields a more effective utilization of the rotor inertia as an energy storage, which is so far untapped by all classical control schemes. NMPC’s advantageous capability to explicitly handle operational constraints can of course be employed to enforce hard limits on the energy storage exploitation.
It is formally shown that an ENMPC formulation can be mimicked by a less insightful tracking NMPC formulation employing wind-speed-dependent, time-varying weights. By introducing an innovative tailored Hessian approximation, this unfavorable transformation can however be avoided, as the seemingly more complex ENMPC problem can be solved with only minor increase in computational load compared to tracking NMPC.
Conclusion
Against common beliefs, economic predictive collective pitch control for wind turbines proves to be real-time feasible, performant and easy to tune. It outperforms classical collective pitch control especially in transient operation phases, which dominate the dynamic turbine behavior under realistic wind conditions. This primarily hinges on the more effective management of the rotor speed. By conducting extensive simulation studies, Pareto-fronts can be generated that aid the design engineer in finding a good balance between power generation and fatigue alleviation.
Learning objectives
The central messages are:
• Economic predictive collective pitch control is real-time feasible on modern embedded control hardware.
• Economically inspired cost functions simplify controller tuning significantly.
• Representative simulation studies using FAST reveal impressive control performance.
Follow EWEA on: