17:00 - 18:30 Annual energy production: improved estimates through advanced modelling
Resource assessment


Room: Hall G2
Advanced modelling methods are now standard in wind estimating modelling of annual energy production (AEP). In this session, speakers will present round robin tests of models for spatial variability of wind resource on projects using different modelling approaches. We will look at the consequences of including atmospheric stability in the calculation of AEP offshore and how you can measure wind profiles at heights of 100-200 metres by using LIDARs with emphasis on charactering extreme shear situations such as low-level jets causing extreme loads. Finally, we will hear about the variability of turbulence intensities measured offshore.
You attended this session?
Learning objectives
- Understand the different methods for modelling the spatial variability of wind resource at the project scale and the errors based on the different modelling approaches;
- How to incorporate stability in the AEP modelling in offshore wind farms;
- Understand the effect of using high resolution modelled wind climatologies combined with advanced boundary modelling on the estimation of the tall winds at 100-200 metres;
- How to characterise and define the extreme events such as low-level jets that can cause fatigue loads in a wind farm;
- Understand the variability of the turbulence intensities offshore measured from multiple measurement towers.
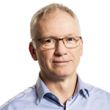
Presenter
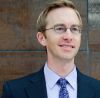
Co-authors:
Matthew Hendrickson (1) F P Isabel Lemos (1)
(1) Vaisala, Seattle, United States
Presenter's biography
Biographies are supplied directly by presenters at WindEurope Summit 2016 and are published here uneditedDr. Eichelberger joined Vaisala in January 2006, and currently serves as Wind Energy Offering Manager. During his tenure at Vaisala, Dr. Eichelberger has been integral in the development, production, and management of Vaisala’s energy assessment capabilities and products. Prior to his time at Vaisala, Dr. Eichelberger was a Research Assistant at the University of Washington in the Department of Atmospheric Sciences. At UW, he conducted research focused on understanding the impacts of climate change on the dynamics of large-scale atmospheric circulations.
Abstract
Round-robin validation of spatial wind modeling at a site with a large number of towers
Introduction
Several recent studies have compared the relative performance of different classes of spatial wind modeling techniques. These classes usually include linear flow models, computational fluid dynamics models, and numerical weather prediction models (usually downscaled with a simpler mass conserving microscale model). Using a unique dataset with a large number (> 15) of met towers across a tropical site of moderately complex terrain, we revisit this intercomparison of modeling approaches. Furthermore, we explore not just the results of the intercomparison, but also the underlying reasons why these three fundamentally different approaches perform differently.
Approach
The spatial mapping of wind resource for the study project is modeled following typical industry procedures for the different modeling approaches. In each case, the goal is to model the wind field using only information provided at a subset of the met masts, as well as site characteristics such as elevation and land use; and then predict the wind speed at towers that were withheld from the modeling, and examine the errors at those locations. For mesoscale modeling, we run the mesoscale numerical weather prediction model with a nested domain configuration, with the innermost grid at the highest resolution of 500 m. The initial conditions and boundary conditions are taken from a global reanalysis dataset. To downscale to the turbine-spacing scale, we apply a mass-conserving wind flow model on a 90-m grid using the 500-m NWP model fields as input. Finally, we develop statistical relationships between the model result and the known tower measurements, and spread those relationships across the project domain to yield wind climatologies at the target (unknown) towers. For the linear flow and CFD models, we run the model using the known tower measurements directly as input for specifying the external flow conditions, and then use the model output directly to predict the wind climate at the target locations.
Main body of abstract
This study is ongoing, and results are not yet finalized. All possible predictor/target tower combinations will be run following the procedure described above, and validation statistics will be compiled. We will look at results such as overall root mean-squared error, absolute errors as a function of distance, absolute errors as a function of intervening terrain complexity, and others. Of particular interest to us is the error behavior with respect to distance. Vaisala’s uncertainty model employs analytic functions to represent the increase in spatial modeling error both close to the met tower (“microscale error”) and at greater distances as the error asymptotes to the typical raw mesoscale model error (“macroscale error”). We will confirm the extent to which these analytic functions are supported by the validation results, and compare how the errors of other modeling approaches, which have no information on spatial variability or gradients of the weather itself, behave at large distances.
Conclusion
Although this study is not yet complete, we anticipate that the size of the dataset (>15 towers) and the analysis performed will yield statistically robust and revealing information about the error behavior of different commonly used spatial wind modeling approaches in the wind energy assessment industry. We’ll be able to compare not just the errors of the different modeling approaches, but how those errors depend on terrain complexity and distance.
Learning objectives
1. Understand the different methods for modeling the spatial variability of wind resource at the project scale.
2. Understand how a round-robin spatial modeling validation study is performed.
3. Understand the behaviors of errors in spatial wind modeling, as a function of distance from the predicting met tower, terrain and land complexity, for different modeling approaches.
Follow EWEA on: